The manufacturing sector quickly embraces Internet of Things (IoT) technologies to enhance productivity, lower expenses, and obtain insights. Among the applications of IoT is the benefits of predictive maintenance, which utilizes sensor data and analytics to anticipate equipment malfunctions in advance. For original equipment manufacturers (OEMs), integrating predictive maintenance capabilities into their products can provide significant economic advantages.
Benefits of Predictive Maintenance in OEM Manufacturing
IoT predictive maintenance allows OEMs to monitor equipment health, predict failures, and schedule proactive repairs. This results in increased uptime, optimized maintenance costs, improved asset utilization, and higher customer satisfaction. Here, we will explore the top 5 economic benefits of predictive maintenance in OEM manufacturing.
The Value of Data: Real-Time Equipment Monitoring
The proliferation of low-cost, networked sensors has unleashed a tsunami of machine data. However, data in itself has little value. To generate the benefits of predictive maintenance, data must be translated into actionable business insights. This is the promise of IoT integration.
OEMs access real-time operational data by connecting critical equipment assets to the Industrial IoT. Streaming telemetry from industrial control systems and onboard sensors provides visibility into equipment health and performance.
Advanced pattern recognition and machine learning algorithms can analyze this data to identify anomalies and detect signs of deterioration. The resulting alerts allow maintenance teams to isolate the root causes of downtime and predict failures before they occur. This enables OEMs to transition from costly reactive repairs to more strategic predictive maintenance programs.
For example, an industrial giant can equip its construction machinery with thousands of sensors that monitor engine RPM, oil pressure, fuel burn, and other metrics. This data can be fed into AI algorithms that track machine health and trigger alerts when abnormalities are detected. By predicting failures, the company can reduce unexpected downtime and repair costs.
Optimized Maintenance Costs: Doing More with Less
Maintenance represents one of the largest cost categories for asset-intensive OEMs. Keeping complex machinery in good working order requires significant technicians, repair parts, diagnostic tools, and shop infrastructure investments. IoT integration introduces new data streams that allow OEMs to optimize maintenance costs in multiple areas.
IoT data also enables maintenance optimization by transitioning technicians from costly “break-fix” repairs to higher-value predictive work. Unplanned downtime is hugely expensive as teams scramble reactively to diagnose and repair crippled assets. Predictive maintenance IoT creates a scheduled workflow, allowing for more efficient labor allocation.
Technicians can be properly staffed, supplied with needed parts, and tasked with higher-skill predictive repairs. This raises productivity while reducing overtime expenses.
Sensor telemetry further optimizes costs by eliminating unnecessary maintenance work. Assets are often serviced based on conservative estimate durations that prematurely restore components. This results in wasted person-hours, needless part replacements, redundant shop time, and lost uptime from extra downtime.
IoT provides granular insights into true component conditions so maintenance can be executed only when required. Resources are focused where they have maximum economic impact.
Finally, IoT enables significant reductions in spare parts inventory. Buffer stocks must be carried out to avoid delays during unplanned failures.
However, predictive maintenance smoothest demand spikes from equipment surprises. IoT data also improves failure pattern analysis so parts needs can be forecasted more accurately. This allows OEMs to “right size” spare parts inventory to balance availability and cost.
Smarter Asset Utilization: Improved Efficiency and Throughput
Unplanned downtime has a direct negative economic impact by reducing productive runtime for OEM machinery and equipment assets. However, the downstream opportunity costs of downtime often far outweigh the immediate production losses.
Business relationships become jeopardized when customers cannot meet their customer commitments due to supplier equipment failures. Furthermore, unexpected downtime frequently causes costly operational disruptions such as overtime, expedited freight, substitute rental equipment, rescheduling maintenance work, etc.
By keeping assets online and avoiding failures, IoT predictive maintenance helps OEMs maximize the utilization of capital-intensive machinery. Productivity increases when reliable uptime meets market demand. This creates throughput headroom to take on new customers and expand market share. Smarter utilization of existing assets also defers costly capital expenditures for new capacity since current production resources can stretch further.
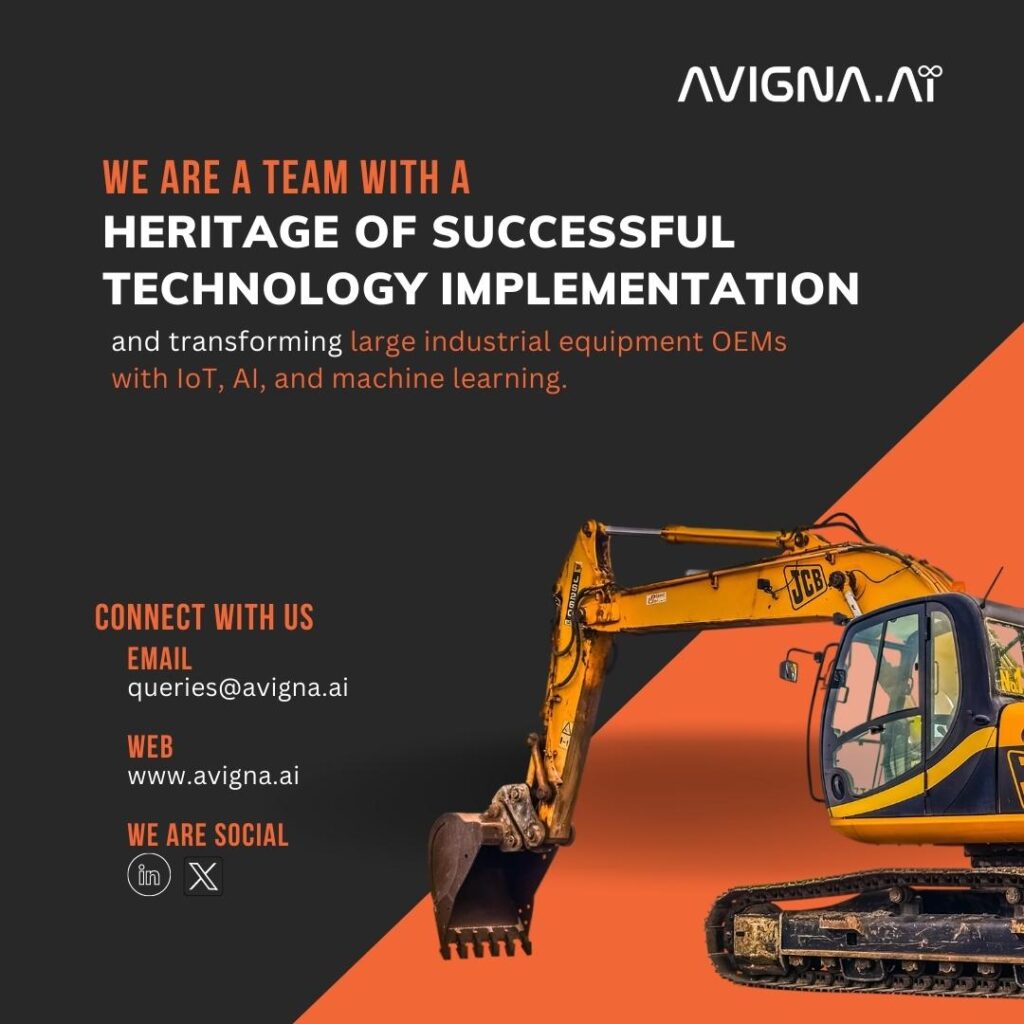
Customer Success: Higher Output & Lower Total Cost of Ownership
While predictive maintenance and optimized asset utilization provide direct economic value to OEMs, IoT integration also enables manufacturers to improve customer success.
For an OEM, customer retention hinges on equipment uptime, performance, and total cost of ownership. IoT delivers insights to advance these key customer-centric metrics.
Firstly, IoT predictive maintenance inherently translates into more reliable uptime for end customers. Manufacturing output ties directly to OEM equipment availability. By keeping assets online through condition monitoring and predictive servicing, OEMs enable customers to meet production targets consistently. This earns customer trust along with the opportunity for expanded machine orders within the installed base.
Next, IoT provides transparency into actual machine utilization and productivity. Customers grapple with determining the optimal throughput capabilities of OEM equipment for their specific operation. IoT data elucidates real-world loading profiles, cycle rates, and performance trends.
Manufacturers can analyze this data to devise tailored solutions that maximize customer throughput, efficiency, and quality.
New Revenue Streams
Integrating predictive maintenance capabilities into equipment opens recurring revenue opportunities for OEMs beyond the initial equipment sale. Value-added services can be sold through performance-based contracts.
OEMs can sell uptime guarantees rather than just warranty coverage for equipment failures. If the asset availability drops below an assured threshold, financial penalties apply.
Predictive maintenance IoT can also be packaged as a subscription service based on equipment utilization hours. Customers only pay for the actual time that assets are operational.
These revenue share models align incentives between the OEM and the customer. The focus shifts from just avoiding failures to maximizing equipment effectiveness.
Conclusion
In today’s highly competitive, lean manufacturing environment, unscheduled downtime can be catastrophic from an operations and financial standpoint. Predictive maintenance IoT prevents outages before they occur, maximizing production throughput. The result is reduced costs, maximized uptime, extended equipment lifespan, lowered inventory, and new revenue streams – all of which contribute directly to the bottom line.
Avigna is on a journey to build a connected world. Connect with us to experience the power of IoT, AI and Machine Learning. Follow us on LinkedIn.